Introduction
In the constantly changing landscape of pharmaceuticals, next-generation technologies like artificial intelligence (AI) have shown significant promise in their ability to help life-changing therapies reach patients faster. In the drug discovery space, AI helps screen millions of potential drugs faster than ever before. In the realm of clinical applications, natural language processing (NLP) technologies assist organizations in distilling vast amounts of clinician text to enhance their comprehension of patient outcomes.
Despite these advancements, the application of AI in manufacturing remains significantly underserved. Even at the forefront of digitalization with modern cloud infrastructure that permeates most modern pharmaceutical organizations, there is a lack of data-driven decision-making and modern tools in both the pilot and commercial-scale plants that drug manufacturers rely on to ensure each dose of a therapy brought to patients is of the highest quality and can be delivered in the quickest timeline possible.
This article aims to unravel the current state of AI in pharmaceutical manufacturing, emphasizing successful applications and the potential for these technologies to redefine the industry. With a focus on trends and the near-term outlook, we will explore how these technologies are revolutionizing the development and delivery of pharmaceuticals.
The Current Landscape of AI in Pharmaceutical Manufacturing
AI-Enabled Technologies Shaping Digital Manufacturing
In the realm of pharmaceutical manufacturing, the incorporation of various types of AI has become instrumental in advancing operational capabilities.1
With tools like GPT and Bard, Generative AI emerges as a transformative tool across the pharmaceutical manufacturing value chain. Modern “AI copilots” empower engineers to transform plain language queries into a variety of outputs, enabling the automatic generation of code for diverse tasks such as data transformation, modeling, and optimization. With the most advanced AI copilots, engineers can ask: “Write me an objective function to maximize yield while keeping my temperature and pressure constant and energy consumption minimized” and receive an optimized control strategy.
Machine learning is a much more timeless category that leverages algorithms and data to autonomously identify underlying patterns and relationships. In the pharmaceutical sector, machine learning facilitates data-driven decision-making, enabling a more streamlined and adaptive approach to various manufacturing processes, allowing engineers to model and understand what drives key quality attribute outputs. This technology not only enhances efficiency but also opens avenues for continuous improvement based on real-time insights.
Deep learning, a branch of machine learning, utilizes artificial neural networks to analyze complex data. Through these neural networks, deep learning offers an understanding of intricate process data, identifying patterns and finding abnormalities within pharmaceutical manufacturing. Nonetheless, the opaque nature of these algorithms, often referred to as the "black box," 2 raises concerns about transparency in decision-making, particularly in the context of a heavily regulated industry like pharmaceuticals where manufacturers must abide by good manufacturing practices (GMP).
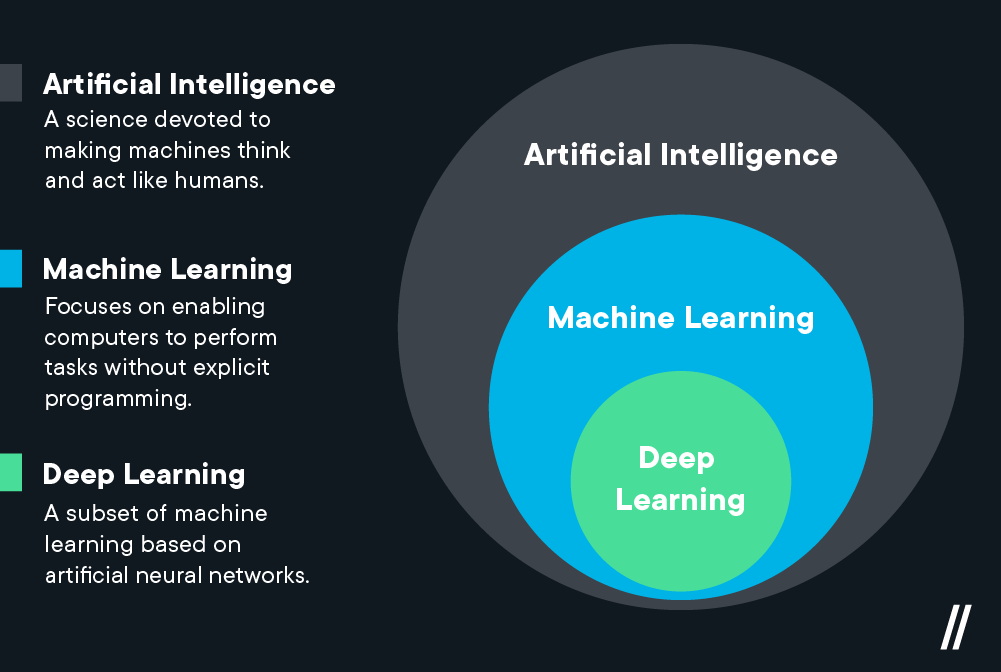
Modern modeling methods such as hybrid modeling represent - as the name would suggest - a middle-ground approach, combining the strengths of traditional first principle models which are fully explainable for regulatory purposes, and data-based machine learning that enable dynamic, real-time decisions. In pharmaceutical manufacturing, this hybrid methodology combines the robustness of established mechanistic models with the context derived from real-world processes and quality data.3
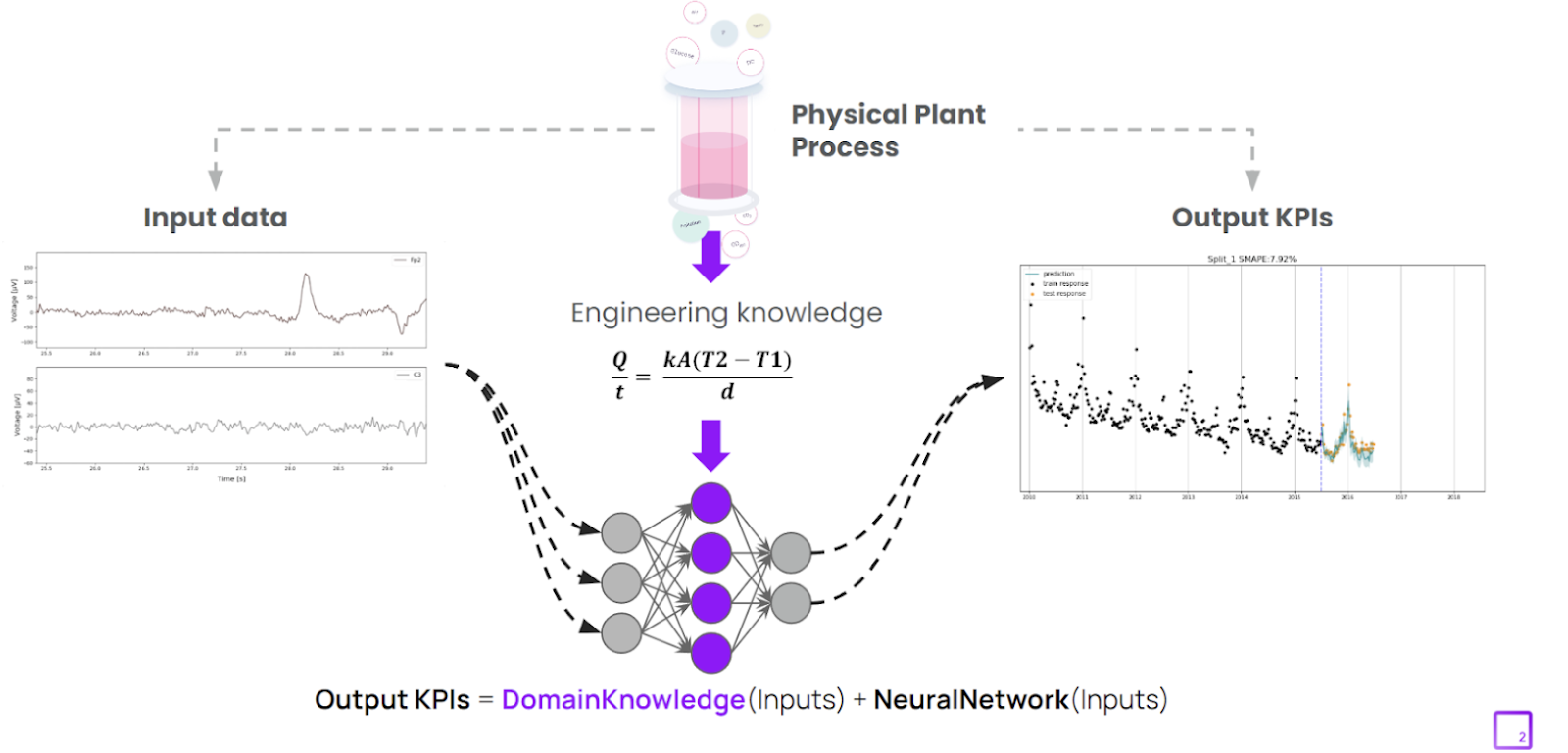
Benefits and Challenges of Implementing AI in the Pharmaceutical Sector
Benefits of Implementing AI
The integration of AI in the pharmaceutical sector holds many benefits in transforming traditional manufacturing processes. The substantial reduction in cycle times is a notable advantage, as AI enables manufacturing teams to streamline existing processes and batch times. This, in turn, brings therapies to the market faster and at a higher quality, while also reducing waste and costs within the production process. The result is an overall improved manufacturing process, visualized and optimized through the use of hybrid modeling and Generative AI.
Beyond efficiency gains, the optimized processes enabled by AI lead to a marked reduction in energy consumption, aligning pharmaceutical manufacturing with sustainable practices. As a cumulative effect, the reduction in cycle times, improved efficiency, and decreased energy consumption collectively contribute to a significant reduction in costs, making AI a cornerstone for economic viability and competitiveness in the pharmaceutical space.4
Challenges Implementing AI
While the benefits of integrating AI in the pharmaceutical sector are substantial, several challenges necessitate careful consideration. The accessibility and quality of existing data may pose a hurdle, as AI systems heavily rely on FAIR datasets (Findable, Accessible, Interoperable, Reusable) for training and decision-making.6 Ensuring the availability of high-quality, relevant data is imperative for the successful implementation of AI in pharmaceutical manufacturing.
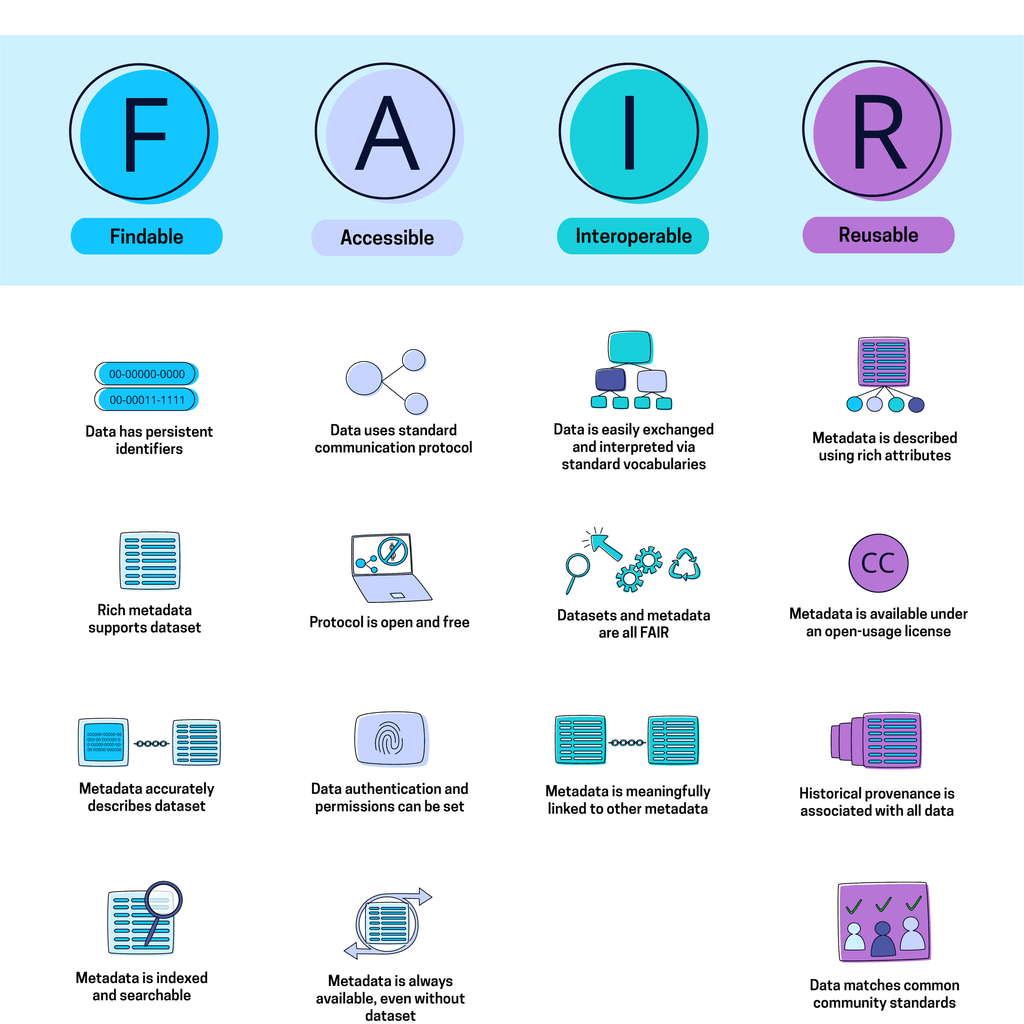
Additionally, there is a need for flexibility in incorporating new technology into organizational structures. This may pose a problem for legacy organizations in navigating existing frameworks, protocols, internal cultures, and other factors to seamlessly integrate AI technologies. Here, a strategic approach to change management and quality-by-design (QbD) is required to foster an environment that embraces the transformative potential of AI, ensuring a harmonious integration that maximizes the benefits while mitigating potential challenges.
Trends Shaping the Future of AI in Pharmaceutical Manufacturing
With projections of the AI in Manufacturing market indicating an explosive surge to $16.3 Billion by 2027 (with a remarkable annual growth rate of 47.9%)7, it is clear that AI is becoming increasingly pervasive in manufacturing. Let's examine several significant trends being leveraged in the field of pharmaceutical manufacturing.
1. Digital Twins in Pharmaceutical Manufacturing
Digital twin technology, in the context of pharmaceuticals, manifests as a virtual mirror of an entire production process or system. For bioprocessing, a digital twin transcends conventional modeling by encompassing the entire process from raw materials to a final product. This virtual representation spans the entire lifecycle of the manufacturing process, continuously updated in real-time through a sophisticated combination of simulation, machine learning, and plant data. The synergy of these elements positions digital twin technology as a powerhouse, driving unparalleled precision, quality, and efficiency in pharmaceutical production.
In the pharmaceutical industry, digital twin technology is harnessed to elevate and streamline existing production processes in real-time. By integrating real-time data and leveraging advanced analytical tools, pharmaceutical manufacturers can gain insights into every facet of the production cycle. This includes optimizing operational parameters, identifying potential bottlenecks, and enhancing overall process efficiency.8
However, implementing digital twins in an industrial, regulated environment can be challenging. From connecting to a variety of data lakes and cleaning data to ensure usability by both humans and machines, all the way to visualization, modeling, and exporting of key model outputs to various stakeholders. For this reason, the successful implementation of digital twins relies on subject matter expertise across multiple teams working synchronously to meet stringent engineering, regulatory, and cybersecurity requirements.9
2. AI-Enabled Quality Control & Safety
Another crucial facet of data analytics lies in process control, where advanced algorithms enhance precision and efficiency. Through AI-driven insights, pharmaceutical manufacturers can optimize various processes, ensuring a more streamlined and adaptable approach. This extends to scale-up optimization, where AI plays a pivotal role in navigating the complexities of increasing production scale while maintaining optimal efficiency.
Equally significant is the impact of AI on safety standards for workers. By leveraging predictive analytics and real-time monitoring, AI fosters a proactive safety culture, identifying and mitigating potential hazards before they pose risks to personnel. This multifaceted integration of AI in pharmaceutical manufacturing not only promises operational excellence but also establishes a foundation for a safer, more agile future in the industry.
3. Lights-out Facilities
Lights-out facilities manufacturing warehouses that run entirely without, or with minimal human involvement.10 In pharmaceuticals, these primarily autonomous environments could result in numerous benefits:
Improving product quality and safety: Lights-out facilities can minimize the risk of product contamination, damage, or mislabeling that may occur with human involvement. This is paramount for a highly regulated industry with critical pharmaceutical products.
Increasing efficiency and speed to market: Fully automated lights-out operations can run 24/7 without breaks, enabling faster production and distribution of medicines.
Energy reduction and safer labor environments: In pharmaceutical facilities containing refrigerated sections, lights-out operations help reduce the traffic going in and out of these areas. This not only cuts down on energy costs and potential damage to goods from increased humidity but also eliminates the need for human labor in harsh, below-zero conditions.11
Regulatory Landscape and Compliance
As AI becomes more prevalent in pharmaceutical manufacturing, companies utilizing AI-enabled technologies will face an increasing need to ensure compliance with industry standards and regulatory requirements.
A robust approach involves transparent documentation and validation of AI algorithms and models, providing a verifiable trail of their accuracy and reliability. This involves not only regular audits but also the incorporation of Quality by Design (QbD) methods. Conducting systematic assessments ensures that AI systems consistently align with existing regulatory frameworks. Additionally, collaborative engagement with regulatory bodies is imperative to enhance understanding and foster acceptance of AI applications in pharmaceutical processes.
The CDER recently published a discussion paper12 to identify areas of policy consideration within AI drug manufacturing. In this paper, they shared how companies using continuously learning AI systems that adapt to real-time data may need to provide clarity on the following areas:
- “The expectations for verification of model lifecycle strategy and the approach for FDA’s examination of continuously updated AI control models during a site inspection, and
- Expectations for establishing product comparability after changes to manufacturing conditions introduced by the AI model, especially for biological products”

The demand for AI explainability has led to the emergence of alternative modeling approaches, incorporating fundamentally explainable components driven by the laws of physics or chemistry, such as hybrid modeling. These approaches serve as a strong complement to traditional data-driven modeling. The outputs of these hybrid models are directly connected to explicit engineering and scientific knowledge and allow stakeholders to “look under the hood” into the model decisions, making model-driven decisions easier to understand and interpret.
By adopting a proactive stance toward compliance, pharmaceutical companies can leverage the transformative power of AI while adhering to the stringent standards that govern the industry, fostering trust and reliability in their operations.
Conclusion
The convergence of AI with pharmaceutical manufacturing illuminates a pathway toward unprecedented efficiency and innovation. While AI has already demonstrated its transformative impact in drug discovery and clinical processes, its full potential within manufacturing remains untapped. Through the integration of AI-enabled technologies, pharmaceutical companies are empowered with data-driven decision-making to quickly bring medicine to patients in clinical trials and ensure that every dose is of the highest quality.
However, implementing AI in this context is not without challenges, demanding a careful balance between technological integration, data governance, and regulatory compliance. With a steadfast commitment to navigating these challenges, pharmaceutical companies can harness the transformative power of AI, fostering a landscape where innovation, compliance, and operational excellence harmoniously coexist within pharmaceutical manufacturing.
References:
- Kulkov I. The role of Artificial Intelligence in Business Transformation: A case of pharmaceutical companies. Technology in Society. June 9, 2021. Accessed January 19, 2024. https://www.sciencedirect.com/science/article/pii/S0160791X21001044.
- University of Michigan, ed. Ai’s mysterious “black box” problem, explained. Dearborn. March 6, 2023. Accessed January 19, 2024. https://umdearborn.edu/news/ais-mysterious-black-box-problem-explained.
- Abdul Gaffoor T. Introduction to hybrid modelling for Digital Twins. Introduction to Hybrid Modelling for Digital Twins. October 7, 2022. Accessed January 19, 2024. https://www.basetwo.ai/blogs/introduction-to-hybrid-modelling-for-digital-twins.
- Salib M. How to improve operational efficiency in manufacturing. How to Improve Operational Efficiency in Manufacturing. August 8, 2023. Accessed January 19, 2024. https://www.basetwo.ai/blogs/how-to-improve-operational-efficiency-in-manufacturing.
- Salib M. 4 steps to achieve manufacturing process optimization in 2024. 4 Steps to Achieve Manufacturing Process Optimization in 2024. November 21, 2023. Accessed January 19, 2024. https://www.basetwo.ai/blogs/4-steps-to-achieve-manufacturing-process-optimization-in-2024.
- Government of Canada SC. Fair Data principles: What is fair? Government of Canada, Statistics Canada. November 18, 2022. Accessed January 19, 2024. https://www.statcan.gc.ca/en/wtc/data-literacy/catalogue/892000062022002.
- Artificial Intelligence in manufacturing market. Market Research Firm. Accessed January 19, 2024. https://www.marketsandmarkets.com/PressReleases/artificial-intelligence-manufacturing.asp.
- Abdul Gaffoor T. Getting started with Digital Twins: An Introduction to TwinOps. Getting Started with Digital Twins: An Introduction to TwinOps. August 30, 2022. Accessed January 19, 2024. https://www.basetwo.ai/blogs/getting-started-with-digital-twins-an-introduction-to-twinops.
- Salib M. Overview of the digital twin lifecycle: A deeper dive into TwinOps. Overview of the Digital Twin Lifecycle: A Deeper Dive Into TwinOps. October 25, 2022. Accessed January 19, 2024. https://www.basetwo.ai/blogs/overview-of-the-digital-twin-lifecycle-a-deeper-dive-into-twinops.
- Mecalux. (n.d.). Lights-out warehouse: The Pinnacle of Industrial Automation. Interlake Mecalux, Warehouse solutions. https://www.interlakemecalux.com/blog/lights-out-warehouse
- What’s a lights-out warehouse and how is automation involved? CertifyMe.net. (2020, March 24). https://www.certifyme.net/osha-blog/whats-lights-out-warehouse/
- Artificial Intelligence in drug manufacturing. March 2023. Accessed January 19, 2024. https://www.fda.gov/media/165743/download?attachment.